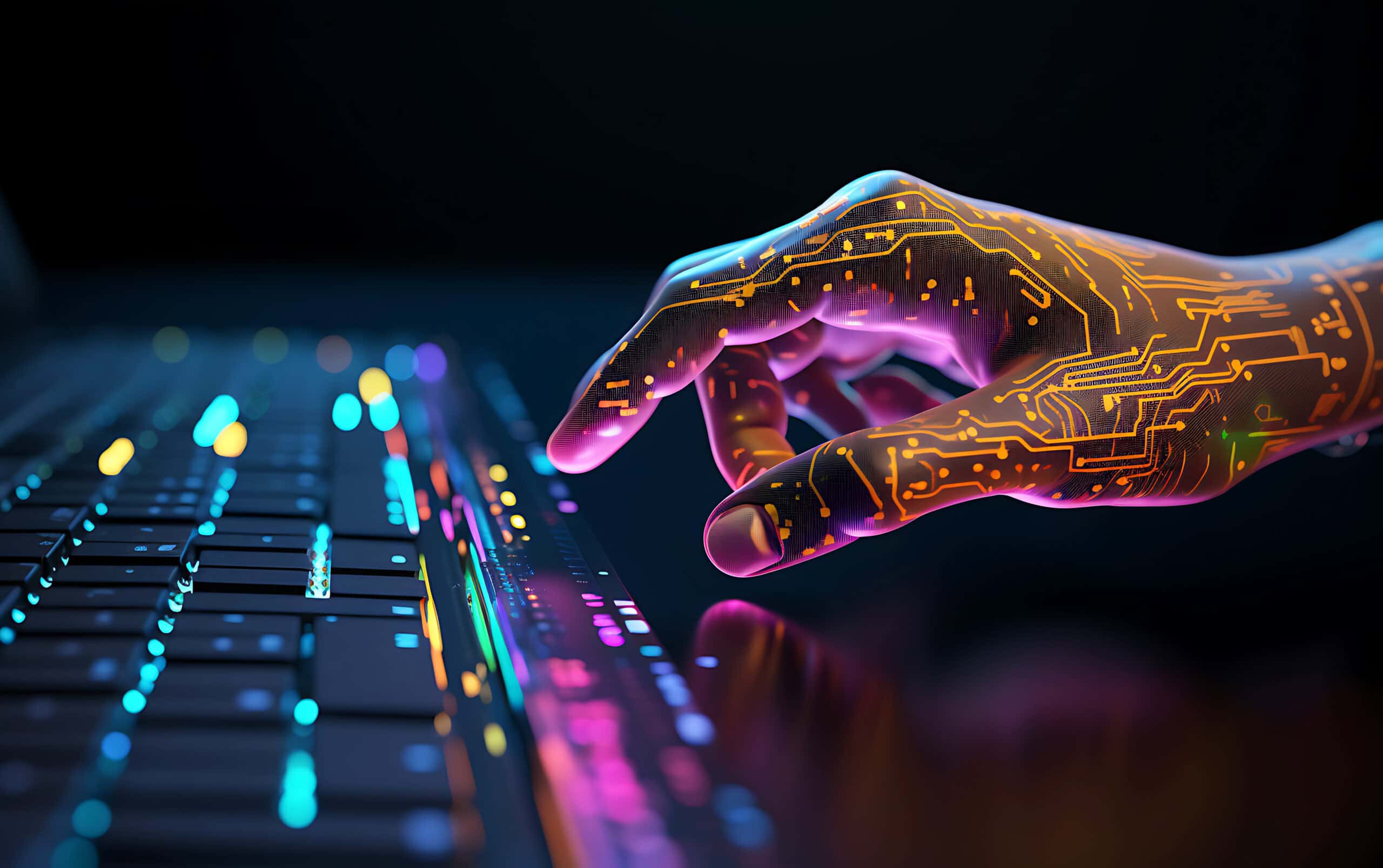
In today’s data-driven world, organizations are inundated with vast amounts of data. The challenge isn’t just about collecting data; it’s about transforming this data into actionable knowledge that can drive strategic decisions.
This transformation process is where Machine Learning (ML) comes into play, providing powerful tools to move from raw data to insightful knowledge. Let’s explore how ML facilitates this transition and why it’s crucial for modern businesses.
The Data-Information-Knowledge Pyramid
Understanding the role of ML in knowledge generation requires us to revisit the classic Data-Information-Knowledge pyramid:
- Data: Raw, unprocessed facts and figures without context. For example, sales numbers, customer feedback, and social media metrics.
- Information: Processed data that provides meaning. When data is organized and analyzed, it becomes information, such as sales trends over time or average customer satisfaction scores.
- Knowledge: Actionable insights derived from information. Knowledge enables decision-makers to understand the ‘why’ behind the data, guiding strategic planning and actions.
From Data to Information
The first step in this journey is converting raw data into useful information. ML algorithms play a pivotal role here by automating data processing tasks, identifying patterns, and highlighting correlations that might not be apparent through manual analysis.
Data Cleaning and Preprocessing
Raw data is often messy and incomplete. ML techniques can automate the data cleaning process, handling missing values, correcting errors, and standardizing formats. This step ensures the data is accurate and reliable for further analysis.
Data Analysis and Pattern Recognition
ML algorithms excel at detecting patterns and trends within large datasets. Techniques such as clustering, classification, and regression analysis help transform raw data into meaningful information. For instance, clustering can group similar customer profiles, while regression analysis can predict future sales based on past performance.
From Information to Knowledge
Once information is extracted from data, the next challenge is turning this information into actionable knowledge. ML goes beyond pattern recognition to provide deeper insights and predictive capabilities.
Predictive Analytics
One of the most powerful applications of ML is predictive analytics. By analyzing historical data, ML models can forecast future trends and behaviors. For example, predictive maintenance in manufacturing uses ML to predict equipment failures before they occur, reducing downtime and saving costs.
Natural Language Processing (NLP)
NLP enables machines to understand and interpret human language. This technology can analyze vast amounts of textual data, such as customer reviews and social media posts, to extract sentiments, opinions, and emerging topics. This information becomes knowledge that companies can use to improve customer satisfaction and tailor their marketing strategies.
Prescriptive Analytics
Prescriptive analytics goes a step further by not only predicting future outcomes but also recommending actions to achieve desired results. ML algorithms analyze various scenarios and suggest optimal decisions. For instance, in supply chain management, ML can recommend the best inventory levels to minimize costs while meeting customer demand.
The Role of ML in Continuous Learning
A key advantage of ML is its ability to continuously learn and adapt. As new data flows in, ML models update and refine their predictions, ensuring the knowledge generated is always current and relevant. This continuous learning process is critical in dynamic environments where conditions change rapidly, such as finance and healthcare.
Real-World Applications of ML in Knowledge Generation
Several industries are already leveraging ML to turn data into knowledge:
Healthcare
ML is revolutionizing healthcare by enabling early disease detection, personalized treatment plans, and efficient patient management. For example, ML models analyze medical records and imaging data to identify patterns indicative of diseases, leading to early interventions and better patient outcomes.
Marketing
Marketers use ML to gain deep insights into customer behavior and preferences. By analyzing data from various touchpoints, ML helps in creating personalized marketing campaigns, optimizing ad spend, and improving customer engagement.
Finance
In finance, ML models predict market trends, assess credit risks, and detect fraudulent activities. By providing accurate and timely insights, ML helps financial institutions make informed decisions, manage risks, and enhance operational efficiency.
Manufacturing
The manufacturing industry stands to gain immensely from the application of ML in knowledge generation. Here are a few key areas where ML is making a significant impact:
Predictive Maintenance
ML algorithms can analyze data from machinery and equipment to predict potential failures before they happen. By identifying patterns and anomalies in sensor data, ML helps in scheduling maintenance activities proactively, thus minimizing unplanned downtime and extending the lifespan of machinery.
Quality Control
In manufacturing, maintaining high product quality is crucial. ML models can process data from various stages of the production line to detect defects and ensure that products meet the required standards. Image recognition and computer vision technologies, powered by ML, can inspect products in real-time, reducing the reliance on manual inspections and increasing accuracy.
Supply Chain Optimization
ML can optimize supply chain operations by analyzing historical and real-time data to forecast demand, manage inventory levels, and streamline logistics. This ensures that manufacturers can meet customer demands efficiently while minimizing costs and avoiding overstock or stockouts.
Production Process Optimization
By analyzing data from production processes, ML can identify bottlenecks and inefficiencies. This enables manufacturers to make data-driven decisions to optimize workflows, enhance productivity, and reduce waste. Process optimization through ML leads to higher efficiency and cost savings.
Energy Management
Energy consumption is a significant cost factor in manufacturing. ML models can analyze energy usage patterns and recommend strategies for reducing energy consumption without compromising production quality. This not only lowers operational costs but also contributes to sustainability goals.
Conclusion
The journey from data to knowledge is a complex but essential process for any organization aiming to stay competitive in today’s fast-paced environment. Machine Learning serves as a powerful catalyst in this journey, automating data processing, uncovering hidden patterns, and generating actionable insights. As ML technology continues to evolve, its role in transforming data into knowledge will only become more critical, driving innovation and efficiency across industries.
In the manufacturing sector, ML is particularly transformative, offering solutions for predictive maintenance, quality control, supply chain optimization, production process improvement, and energy management. These applications demonstrate the vast potential of ML in generating knowledge that leads to smarter, more efficient manufacturing operations.
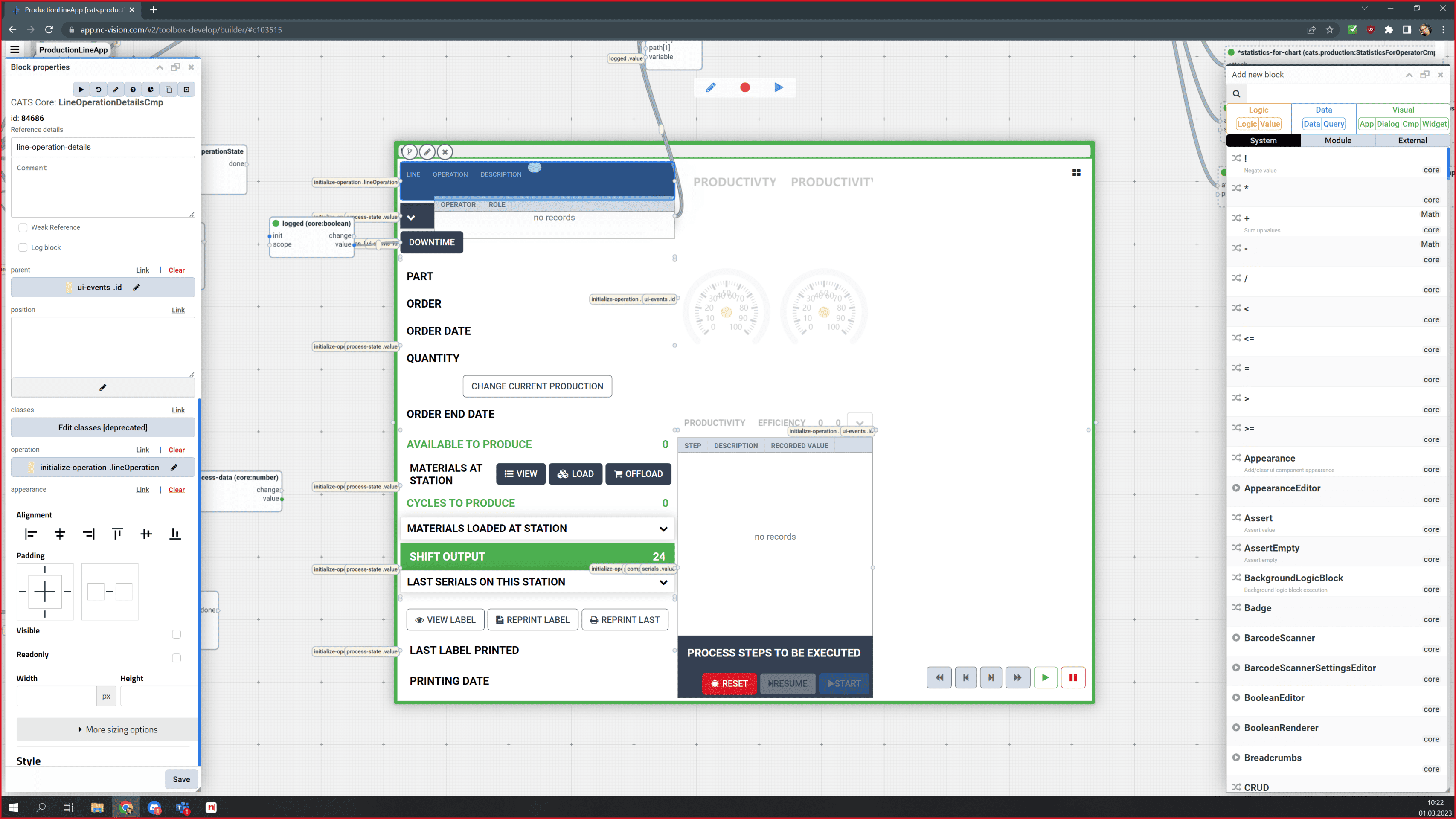
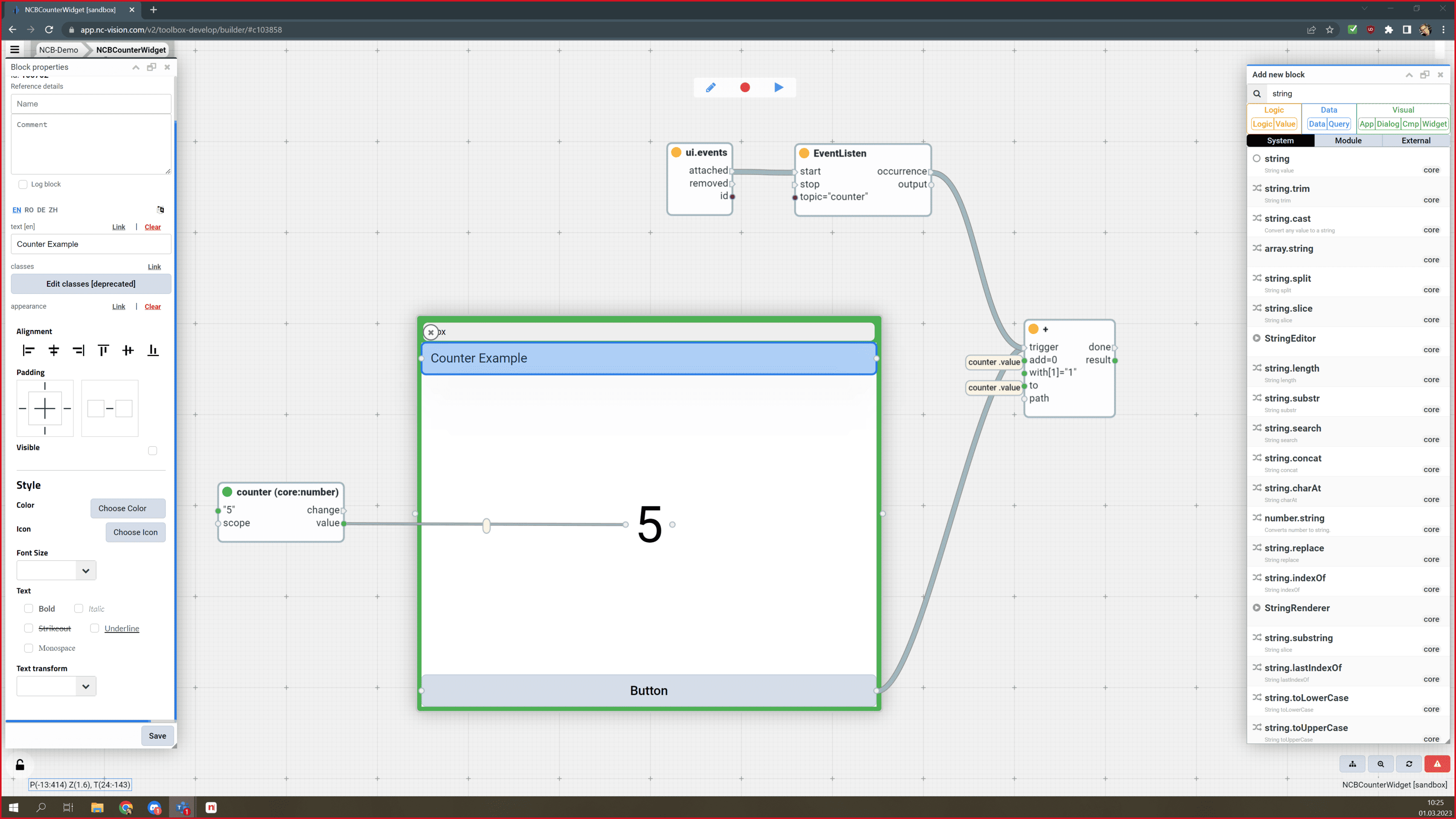
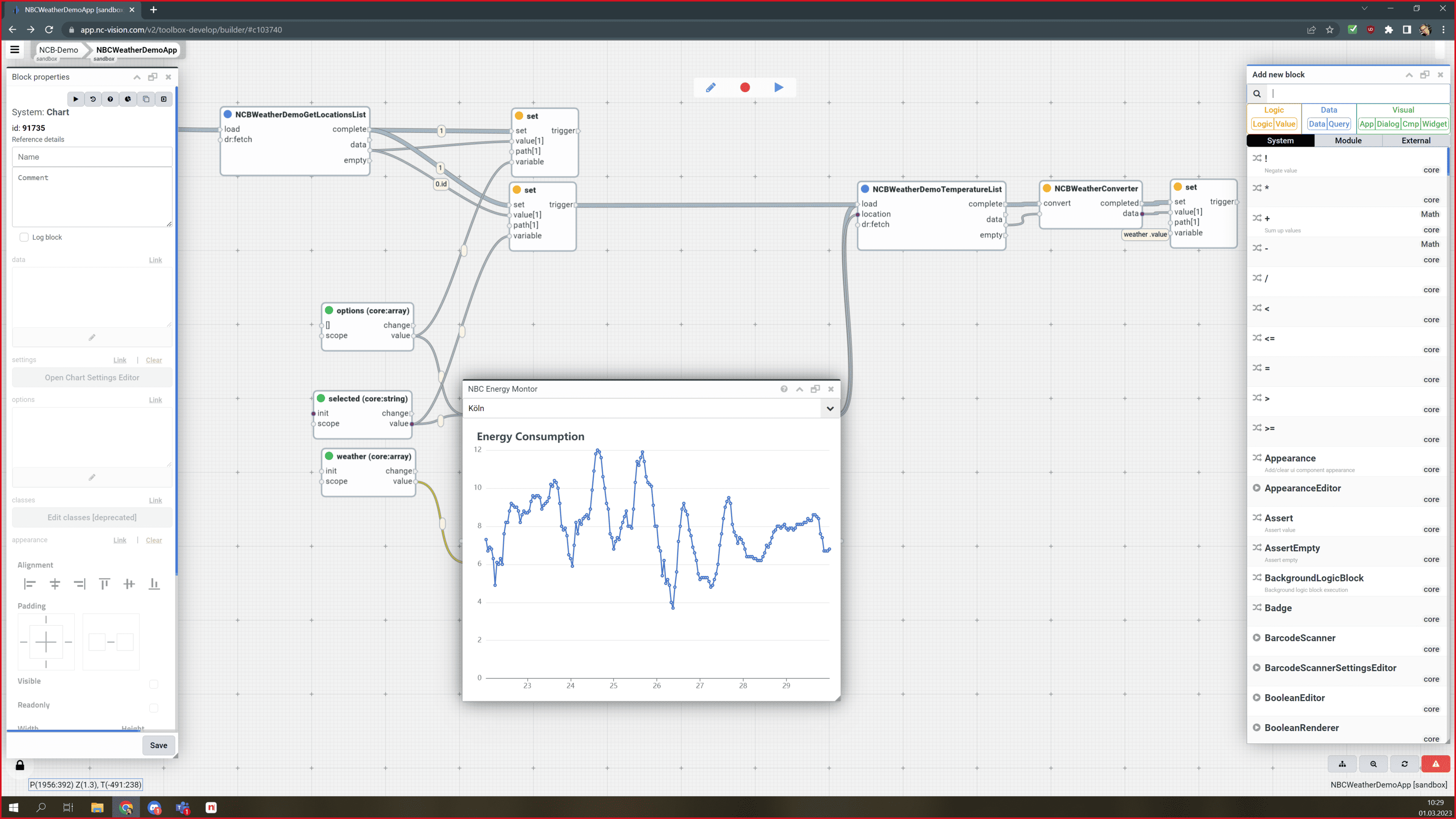
Made for production, manufacturing, and beyond:
Are you ready to harness the power of Machine Learning to generate knowledge and drive your business forward? Explore how our solutions can help you unlock the full potential of your data. Schedule a free platform tour today.
NC-Vision is committed to helping the manufacturing industry make the most of no-code software development. We offer a range of no-code solutions that enable companies to quickly and easily create custom solutions that are tailored to their needs. Our tools allow companies to create solutions faster, more cost-effectively, and with more customization than ever before.