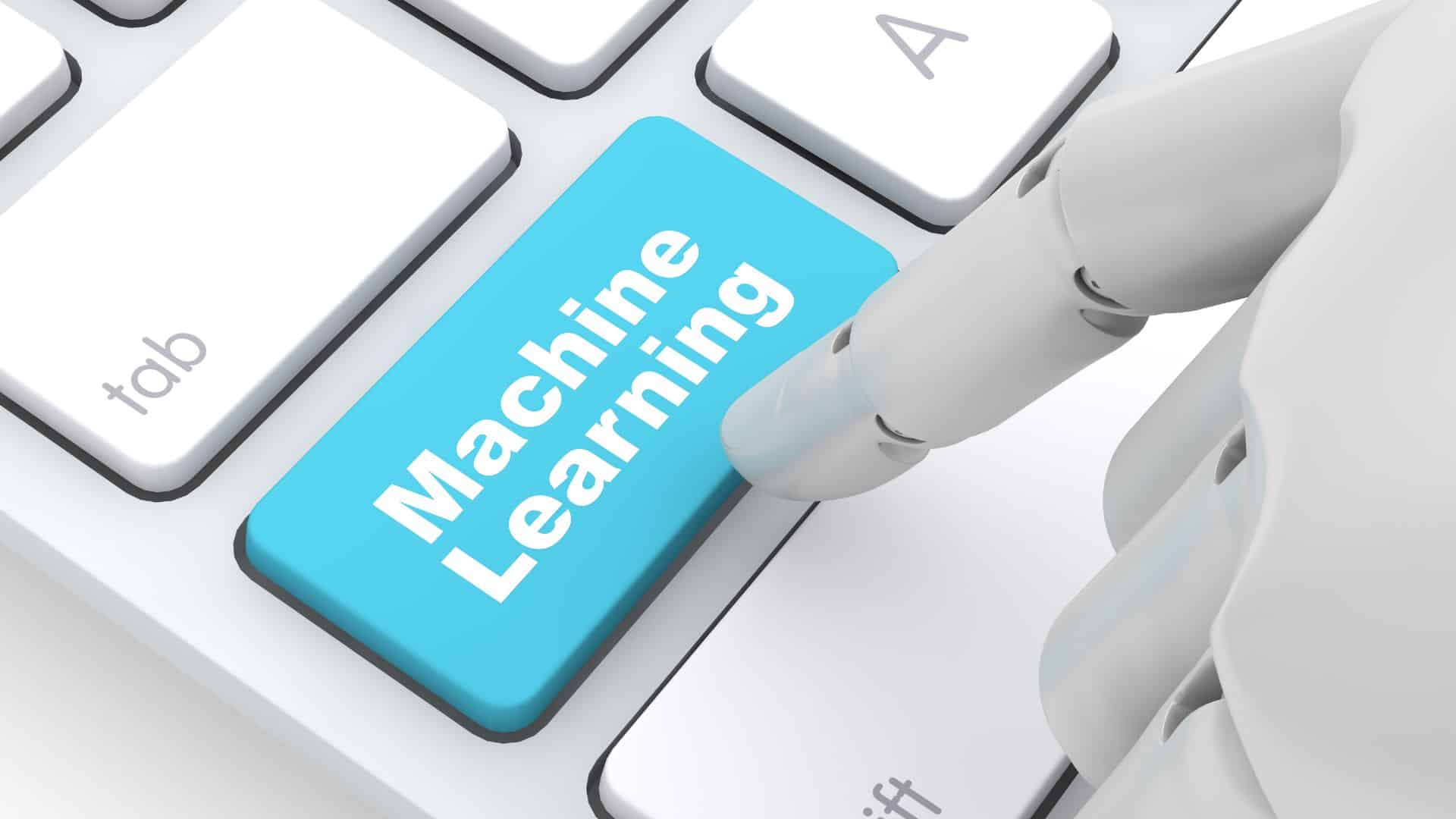
Advancements in technology have opened the door to groundbreaking opportunities in the manufacturing industry, enabling businesses to streamline processes and boost productivity. Among these technological breakthroughs, machine learning stands out as a transformative force, with the potential to revolutionize manufacturing operations. However, its adoption has traditionally been hindered by complexity, requiring expert knowledge and significant resources, leaving many manufacturers unable to take advantage of its immense potential.
Today, a new era is emerging—one that democratizes machine learning in manufacturing. Innovative platforms and tools are making this technology more accessible, empowering manufacturers of all sizes to unlock its capabilities without the need for extensive technical expertise. This shift is breaking down traditional barriers, paving the way for operational excellence and enhanced competitiveness.
In this eBook, we will explore the significance of democratizing machine learning, uncover the benefits and challenges of its implementation, and share inspiring success stories of manufacturers who have embraced this game-changing technology.
Ready to unlock the power of machine learning for your manufacturing operations? Download our eBook, and discover how no-code platforms are transforming the way manufacturers innovate, improve efficiency, and stay competitive. Get your copy now and start your journey toward operational excellence today!
The Importance of Democratizing Machine Learning
In today’s fast-paced manufacturing landscape, the importance of democratizing machine learning cannot be overstated. Traditionally, machine learning has been confined to those with advanced degrees in data science or computer programming, which has left many manufacturers at a disadvantage. By democratizing this technology, businesses of all sizes can harness its capabilities to improve operational efficiency, reduce costs, and enhance product quality. This newfound accessibility means that even small manufacturers can leverage machine learning to gain a competitive edge, allowing them to innovate and thrive in an increasingly complex industry.
Democratization leads to a more inclusive approach to technology adoption, where employees at various skill levels can contribute to the implementation and optimization of machine learning solutions. This empowerment not only boosts morale but also fosters a culture of continuous improvement and innovation. When operators, engineers, and managers are equipped with the tools and knowledge to utilize machine learning, they can make data-driven decisions that enhance productivity and efficiency. Consequently, organizations can respond more swiftly to market demands and shifts, positioning themselves as agile players in the manufacturing sector.
Moreover, democratizing machine learning encourages collaboration among teams, breaking down silos that often exist within organizations. As various departments come together to share insights and data, the synergies created can lead to more comprehensive and effective machine learning applications. This collaboration can manifest in numerous ways, such as predictive maintenance, quality control, and supply chain optimization. By leveraging collective expertise and diverse perspectives, manufacturers can unlock the full potential of machine learning and drive significant advancements in their operational processes.
Challenges in Implementing Machine Learning in Manufacturing
Despite the clear advantages of machine learning, many manufacturers face significant challenges when implementing these technologies. One of the primary hurdles is the lack of skilled personnel who understand both the manufacturing processes and the intricacies of machine learning. Many organizations struggle to find data scientists who can translate complex algorithms into actionable insights that apply directly to manufacturing contexts. This skills gap often results in stalled projects and underutilized technologies, limiting the potential benefits of machine learning.
Another challenge is the integration of machine learning solutions with existing systems and processes. Many manufacturers operate legacy systems that may not easily accommodate new technologies or require significant modifications to do so. This integration issue can lead to increased costs, extended timelines, and operational disruptions, causing organizations to hesitate before embarking on machine learning initiatives. Furthermore, the need for high-quality, consistent data is critical; without it, machine learning models may produce inaccurate predictions, undermining trust in the technology.
Cultural resistance is another barrier that manufacturers must overcome when adopting machine learning. Employees may fear that automation and machine learning will replace their jobs, leading to resistance against new initiatives. Additionally, there may be skepticism about the effectiveness of machine learning, especially in environments where traditional methods have been the norm for decades.
Addressing these concerns requires a proactive approach that emphasizes the complementary nature of machine learning to human expertise, highlighting how it can augment decision-making rather than replace it.
Overcoming Barriers to Democratizing Machine Learning
To successfully democratize machine learning in manufacturing, organizations must take deliberate steps to overcome existing barriers. First and foremost, investing in training and education is essential. By offering programs that enable employees to develop skills in data analysis and machine learning, organizations can cultivate a workforce that is well-equipped to leverage these technologies. Workshops, online courses, and partnerships with educational institutions can help bridge the skills gap and create a culture of continuous learning.
Furthermore, manufacturers should prioritize the development of user-friendly tools that simplify the machine learning process. By creating intuitive platforms that require minimal technical expertise, businesses can empower a broader range of employees to engage with machine learning. These tools often come with built-in templates and guided workflows that facilitate the implementation of machine learning solutions, making it easier for teams to adopt and adapt the technology to their specific needs.
Finally, fostering a culture of collaboration and innovation is crucial for overcoming resistance to machine learning adoption. Encouraging cross-functional teams to work together on machine learning projects can help dispel fears and misconceptions while showcasing the benefits of the technology. Leaders should actively promote success stories and highlight the positive impacts of machine learning on operational efficiency and product quality. By demonstrating the tangible value of machine learning, organizations can create buy-in from all levels of the workforce and pave the way for smoother implementation.
Steps to Get Started with Machine Learning in Manufacturing
For manufacturers looking to embark on their machine learning journey, following a structured approach can streamline the process and enhance the likelihood of success. The first step is to define the specific problem or opportunity that machine learning can address. This could involve identifying inefficiencies in production, quality control issues, or supply chain optimization. By clearly articulating the objective, organizations can focus their efforts on developing solutions that deliver tangible results.
Once the problem has been identified, the next step is to gather and preprocess the relevant data. High-quality data is the foundation of effective machine learning models, so manufacturers must invest time in ensuring that the data is accurate, complete, and formatted correctly. This may involve collecting data from various sources, cleaning it to remove inconsistencies, and transforming it into a suitable format for analysis.
After preparing the data, manufacturers can begin selecting appropriate machine learning algorithms and tools. Depending on the complexity of the problem, organizations may choose to use basic algorithms or explore more advanced techniques such as deep learning. Utilizing user-friendly platforms can significantly expedite this process, allowing teams to experiment with different models and configurations without extensive coding knowledge. Finally, continuous monitoring and iteration are crucial; as new data becomes available, models should be updated and refined to maintain accuracy and relevance.
Best Practices for Implementing Machine Learning in Manufacturing
Implementing machine learning in manufacturing requires careful consideration of best practices to ensure successful outcomes. One of the fundamental best practices is to foster collaboration between data scientists and domain experts. While data scientists possess the technical skills to develop algorithms, domain experts understand the nuances of the manufacturing processes. By working together, these teams can create models that are not only technically sound but also practically applicable to real-world scenarios.
Another important practice is to start small and scale gradually. Manufacturers should begin with pilot projects that address specific challenges, allowing them to test the waters and learn from initial implementations. These early successes can serve as a proof of concept, generating buy-in from stakeholders and paving the way for larger-scale machine learning initiatives. As organizations gain confidence and experience, they can expand their efforts to tackle more complex problems.
Lastly, maintaining an agile mindset is crucial in the fast-evolving landscape of machine learning. Manufacturers should be open to experimenting with new algorithms, tools, and methodologies as the technology continues to advance. Regularly reviewing and updating machine learning models based on performance metrics and changing business needs will help organizations stay ahead of the curve and ensure that their machine learning initiatives remain effective and relevant.
Future Trends in Democratizing Machine Learning for Manufacturing Excellence
As technology continues to evolve, several trends are emerging that will further democratize machine learning in the manufacturing sector. One significant trend is the rise of low-code and no-code platforms, which empower non-technical users to build and deploy machine learning models with minimal coding knowledge. This democratization will allow a broader range of employees to engage with machine learning, fostering innovation and creativity across organizations.
Moreover, the integration of artificial intelligence (AI) with machine learning is expected to drive significant advancements in manufacturing. AI-powered tools will not only automate routine tasks but also enhance decision-making processes by providing deeper insights and predictive capabilities. As these technologies become more accessible, manufacturers will be able to leverage them to optimize operations and improve overall efficiency.
Finally, the growing emphasis on data privacy and security will shape the future of machine learning in manufacturing. As organizations increasingly rely on data-driven insights, the importance of safeguarding sensitive information will become paramount. Manufacturers will need to adopt robust data governance frameworks and ensure compliance with regulations while still harnessing the power of machine learning. This focus on ethical data use will be essential for maintaining trust with customers and stakeholders as the industry continues to evolve.
Conclusion
The democratization of machine learning in manufacturing represents a transformative shift that empowers businesses of all sizes to enhance their operations and achieve excellence. By breaking down barriers and providing accessible tools and resources, manufacturers can leverage machine learning to drive efficiency, improve product quality, and stay competitive in a rapidly changing landscape. While challenges remain, proactive strategies can help organizations overcome these obstacles and fully embrace the potential of machine learning.
As more manufacturers embark on their machine learning journeys, the success stories emerging from the industry will serve as an inspiration for others. By learning from these experiences and adopting best practices, organizations can position themselves to thrive in an era defined by technological innovation.
As we look to the future, the continued democratization of machine learning will undoubtedly play a vital role in shaping the manufacturing sector, paving the way for unprecedented levels of operational excellence and growth.
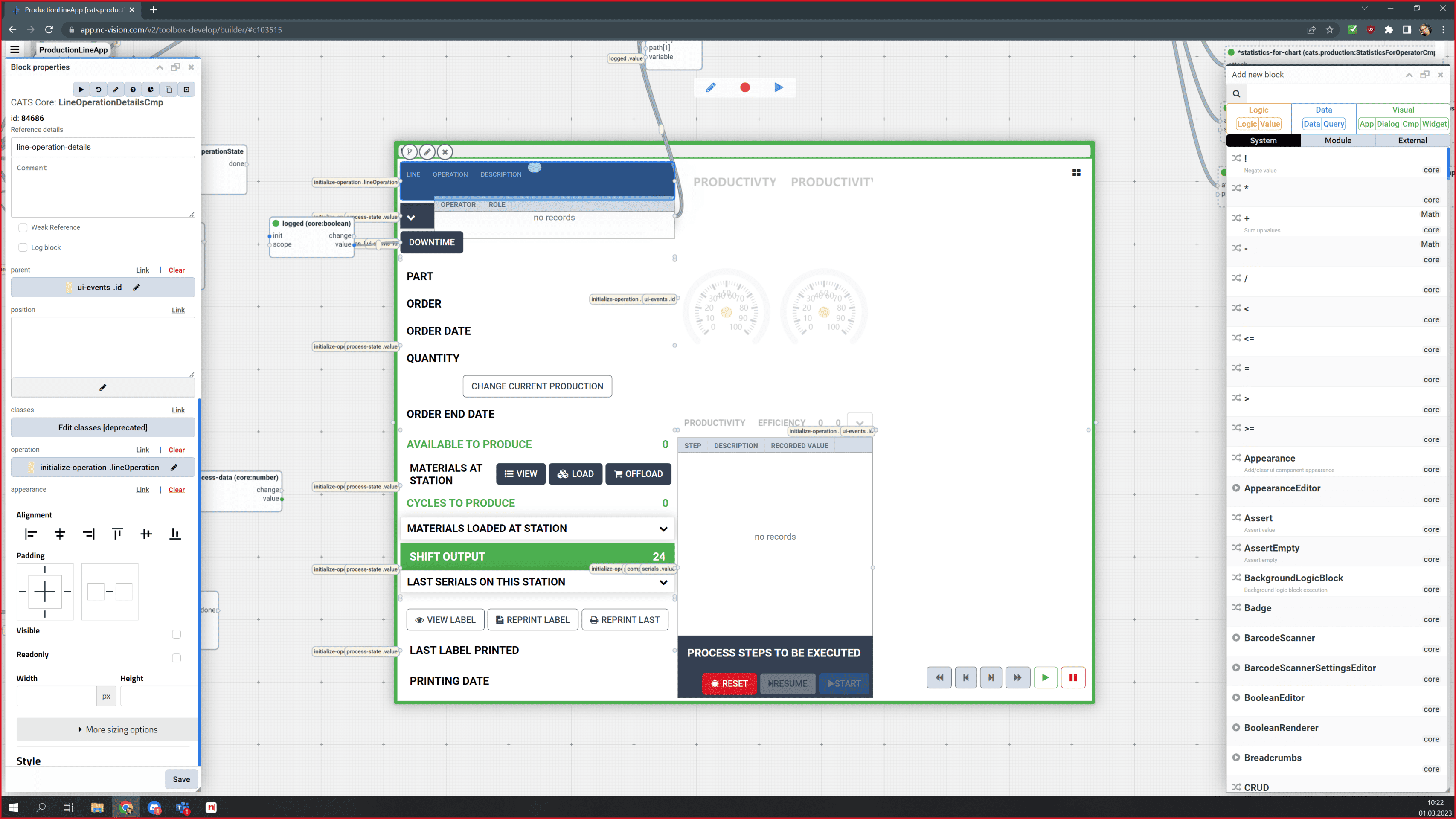
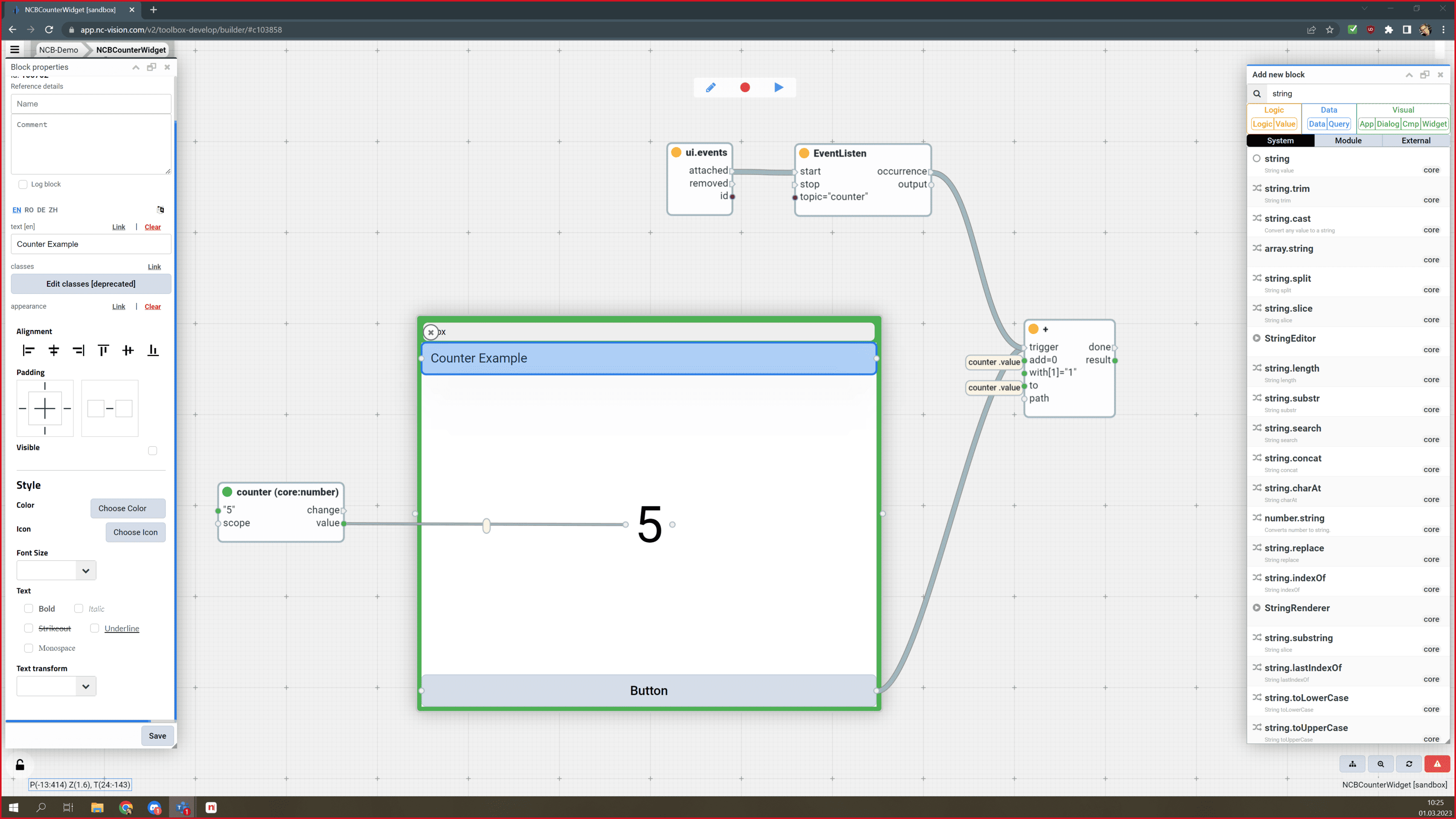
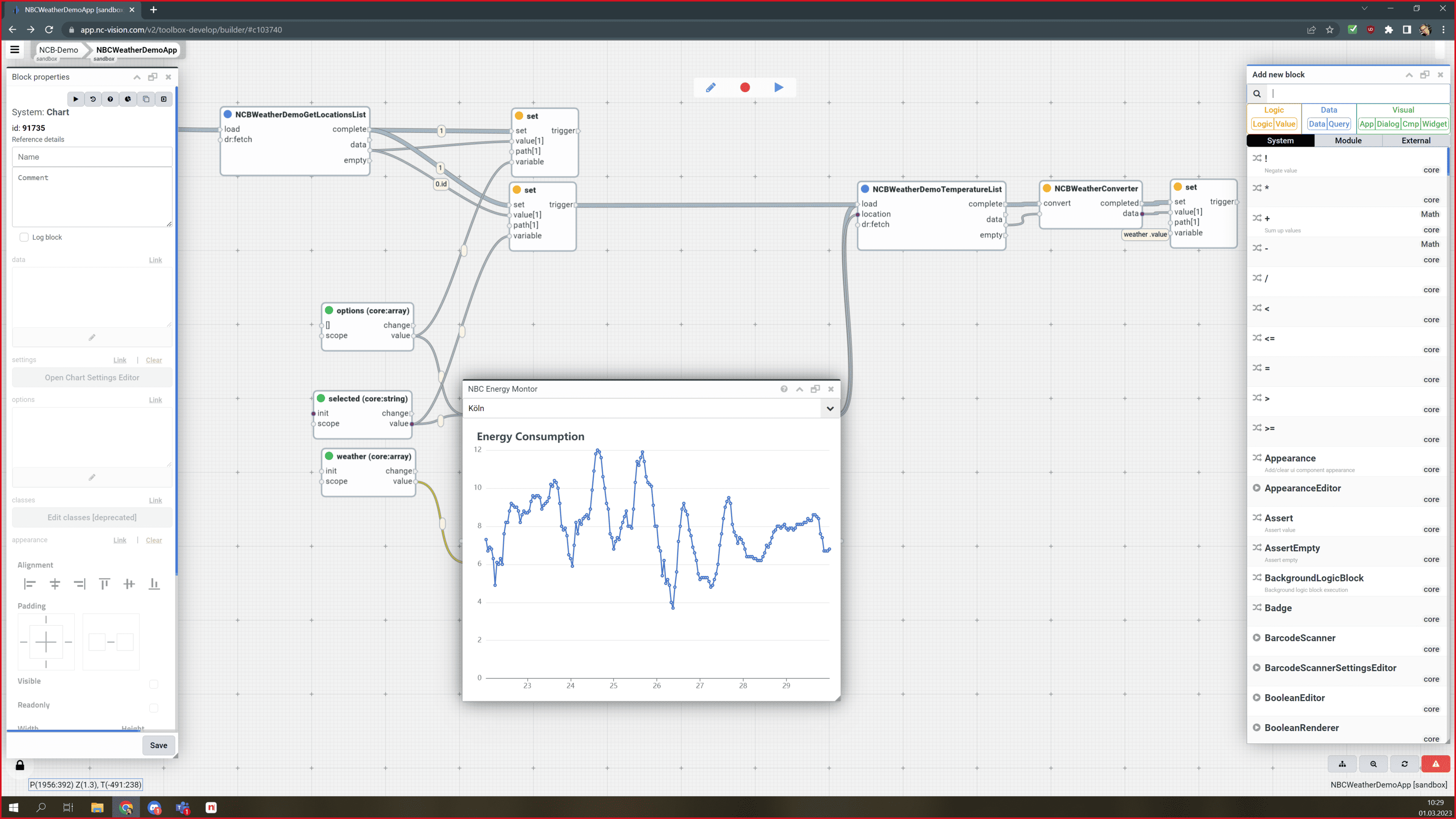
Made for production, manufacturing, and beyond:
Unlock the full potential of machine learning and enhance your manufacturing processes today. Get started with NC-Vision’s no-code platform** and transform your operations—no coding expertise required! Contact us now for a free platform tour and take the first step towards smarter, more efficient manufacturing.
NC-Vision is committed to helping the manufacturing industry make the most of no-code software development. We offer a range of no-code solutions that enable companies to quickly and easily create custom solutions that are tailored to their needs. Our tools allow companies to create solutions faster, more cost-effectively, and with more customization than ever before.