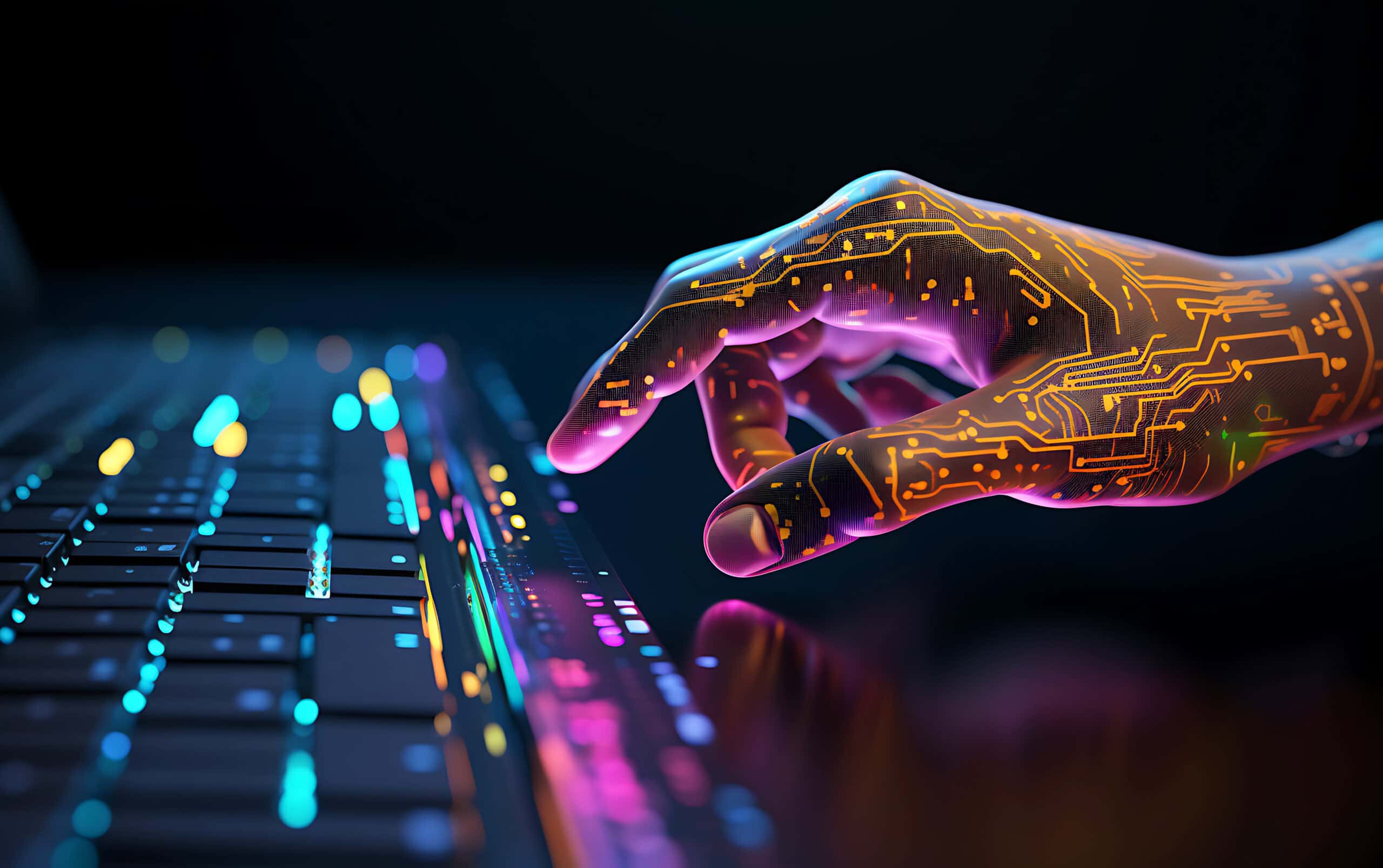
In the ever-evolving landscape of manufacturing, machine learning (ML) has emerged as a game-changer, offering solutions to various production challenges.
The NC-Vision Machine Learning Studio, with its focus on democratizing access to ML and AI, has developed several innovative use cases that streamline production processes, enhance quality control, and optimize resource utilization.
This blog post delves into the key ML use cases in manufacturing, highlighting their impact on efficiency, safety, and sustainability.
The main goal of NC-Vision’s Machine Learning Studio is to bring shopfloor and Machine Learning expertise together. We thus democratize access to ML and AI, making these powerful technologies accessible to users without requiring extensive technical knowledge in data science.
Predictive Maintenance and Real-Time Monitoring of Machinery
Problem: Unexpected Downtime
Industrial machinery often requires maintenance to prevent unexpected downtime, which can significantly disrupt production. However, manual monitoring of machine conditions is not feasible on a large scale.
Solution: Predictive Maintenance Model
A predictive maintenance model using sensor data (e.g., vibration, temperature, pressure) predicts when machinery components are likely to fail. This data is processed in real-time to trigger alerts before failures occur.
The dashboard/kiosk display provides operators with an easy-to-read status of each machine, recommended maintenance actions, and the likelihood of future issues. Alerts can be color-coded, allowing immediate action when required.
Quality Control in Production Using Computer Vision
Problem: Human Error in Quality Inspection
Manually inspecting products for defects is time-consuming and prone to human error.
Solution: Image Classification & Object Detection Model
An image classification model classifies products as either “defective” or “pass” based on images taken from cameras installed along the production line.
Combined with the classification model, object detection identifies specific types of defects (e.g., scratches, dents, misalignments) on products.
Terminals at the production site display flagged products with visual markers, indicating the specific defects. Operators can review the flagged images directly on the kiosks and take corrective actions. The application can send automatic notifications for re-inspection or halting production if a significant number of defects are found.
Automated Product Tracking and Fault Detection in Assembly Lines
Problem: Tracking Product Assembly Progress
Keeping track of product assembly progress and detecting issues at various stages is challenging without automation.
Solution: Object Tracking & Fault Detection Model
This model tracks the movement of products along the assembly line, ensuring that each product follows the correct workflow.
The terminal display for operators – on kiosks along the production floor- shows the current status of the assembly line, with alerts for any products that need rework or inspection. The system visualizes product flow and indicates bottlenecks or failures.
The Fault Detection Model continuously monitors the assembly process and detects deviations from standard operations (e.g., missing components, improper assembly).
Energy Efficiency and Load Balancing in Production
Problem: High Energy Costs and Inefficiencies
High energy costs and uneven power distribution across machines lead to inefficiencies in production.
Solution: Energy Consumption Optimization Model & Demand Forcasting Model
An energy consumption optimization model monitors the energy consumption of each machine and optimizes their usage to reduce power consumption during peak energy cost periods.
Real-time energy usage data and optimization recommendations are shown on kiosks placed across the factory floor. Operators can adjust machine schedules directly based on the displayed recommendations.
The demand forecasting model predicts future energy demand based on current production loads and schedules machines to operate during low-cost periods.
Real-Time Safety Monitoring and Hazard Detection
Problem: Ensuring Safety in Production Facilities
Ensuring safety in a production facility requires constant monitoring of equipment and environmental conditions.
Solution: Anomaly Detection Model for Safety & Computer Vision Model
An anomaly detection model monitors environmental data (e.g., air quality, temperature, humidity) and machine performance data (e.g. pressure levels, noise) to detect hazardous conditions.
The display for operators and managers – terminals around the facility – provides a real-time safety dashboard highlighting hazardous zones or unsafe behaviors. The interface gives immediate instructions for rectifying the situation and sends alerts to safety personnel.
The computer vision model processes live video feeds to detect unsafe actions (e.g., workers without safety gear, blocked emergency exits) and raises alerts.
Smart Production Line Management with Predictive Analytics
Problem: Managing Production Bottlenecks
Efficiently managing production lines requires anticipating production bottlenecks and avoiding delays.
Solution: Production Scheduling Model
The production scheduling model uses historical production data to forecast potential delays or bottlenecks in the production line, adjusting schedules in real-time to optimize throughput.
Production line supervisors can monitor production progress on terminals, where real-time alerts suggest adjustments to production schedules or shifts to alleviate bottlenecks.
Integrated with the scheduling model, a predictive demand model ensures that materials are available in time, reducing delays due to material shortages.
Automated Waste Reduction and Recycling in Manufacturing
Problem: Reducing Waste in Manufacturing Processes
Manufacturing processes often produce significant waste, which leads to increased costs and environmental impact.
Solution: Waste Prediction Model and Material Sorting Model
The machine learning model that analyzes production data predicts the amount of waste generated at different stages of manufacturing.
Terminals placed near waste disposal points provide real-time updates on waste levels, recyclability, and suggested optimizations to reduce material waste. Workers receive step-by-step guidance on recycling or disposing of waste.
Material Sorting Model: A computer vision model identifies recyclable and non-recyclable waste on the production line, sorting materials accordingly for efficient disposal or reuse.
Automated Supply Chain Management and Optimization
Problem: Supply Chain Challenges
Manufacturing facilities commonly encounter supply chain management issues such as delays, inventory mismanagement, and inefficient resource use.
Solution: Demand Forcasting Model and Inventory Management Model
The demand forcasting model predicts future demand for raw materials or components based on historical data, current production levels, and market trends.
Terminal displays for supply chain managers show current inventory levels, predicted demand, and supply order statuses. The interface allows users to adjust orders manually if necessary, or they can let the automated system handle everything.
Inventory Management Model is a machine learning model that ensures optimal inventory levels by automatically replenishing supplies as they are consumed on the production line.
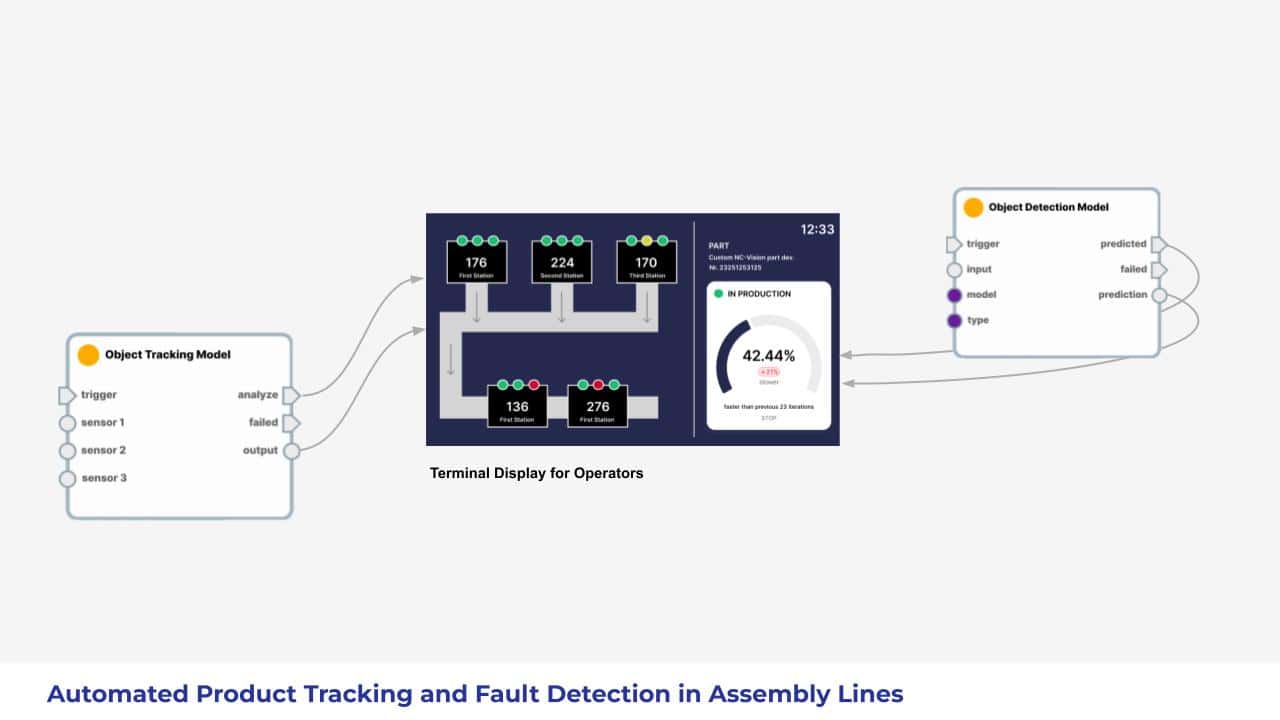
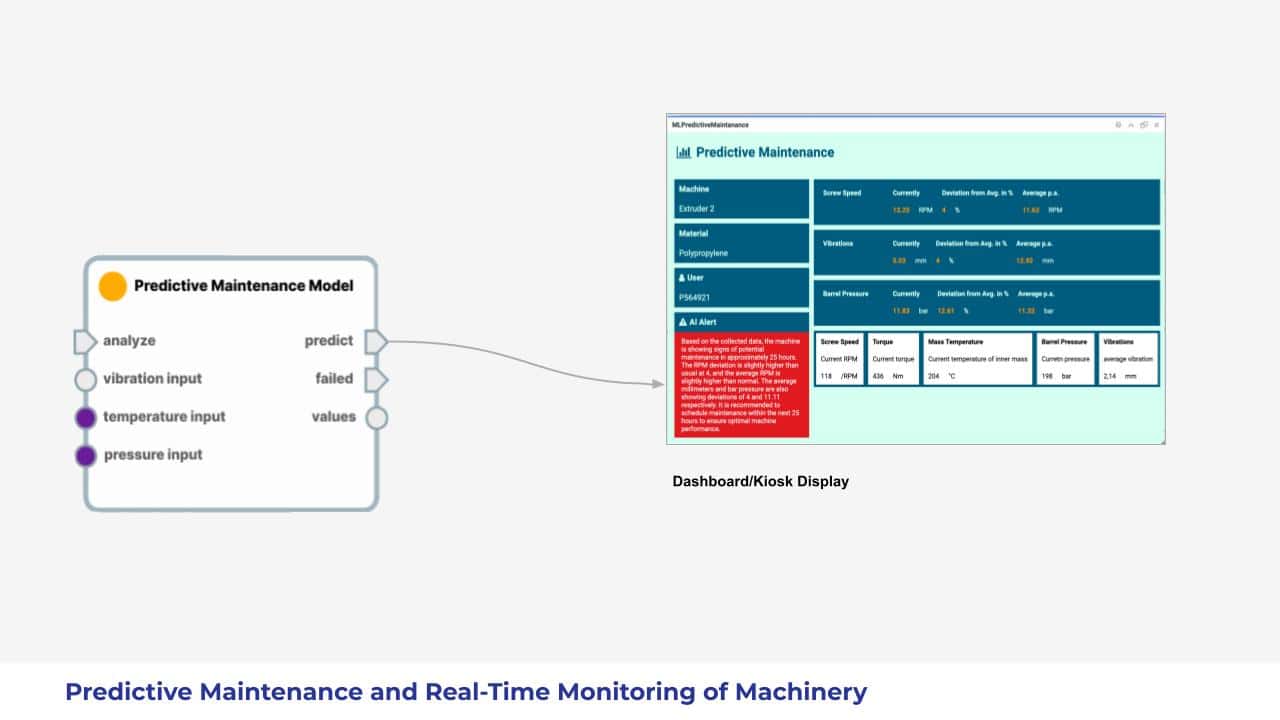
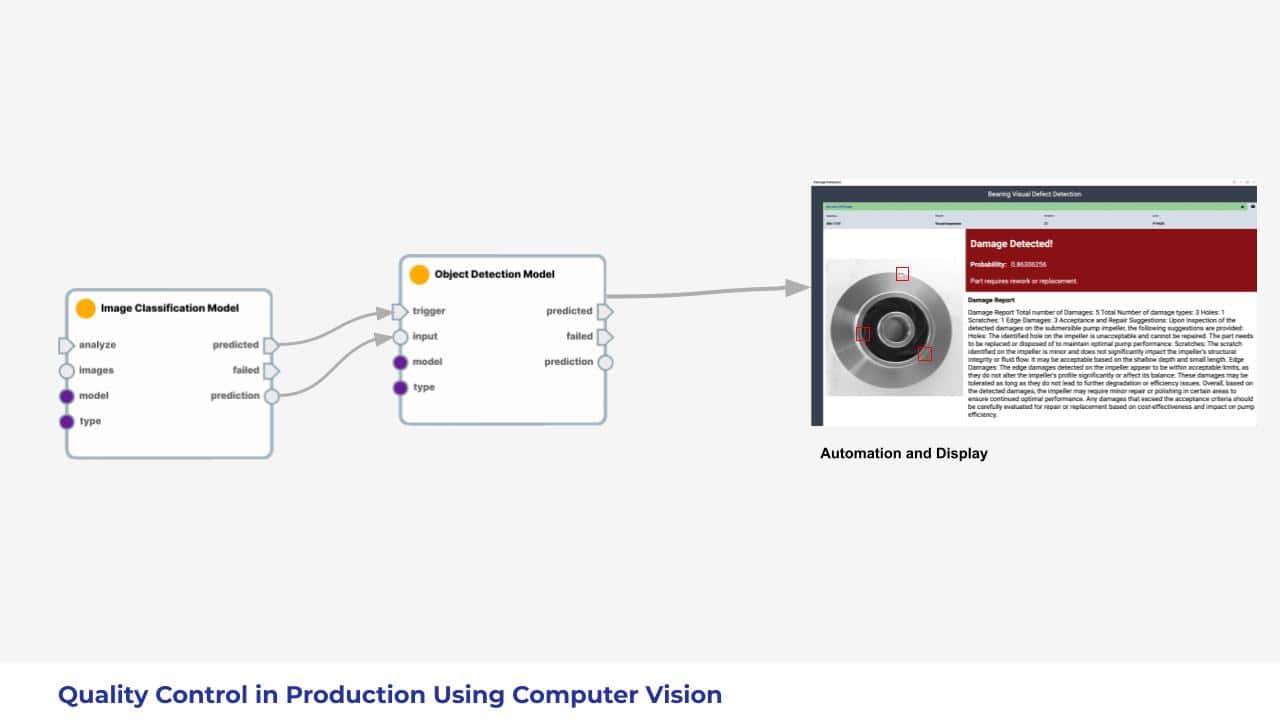
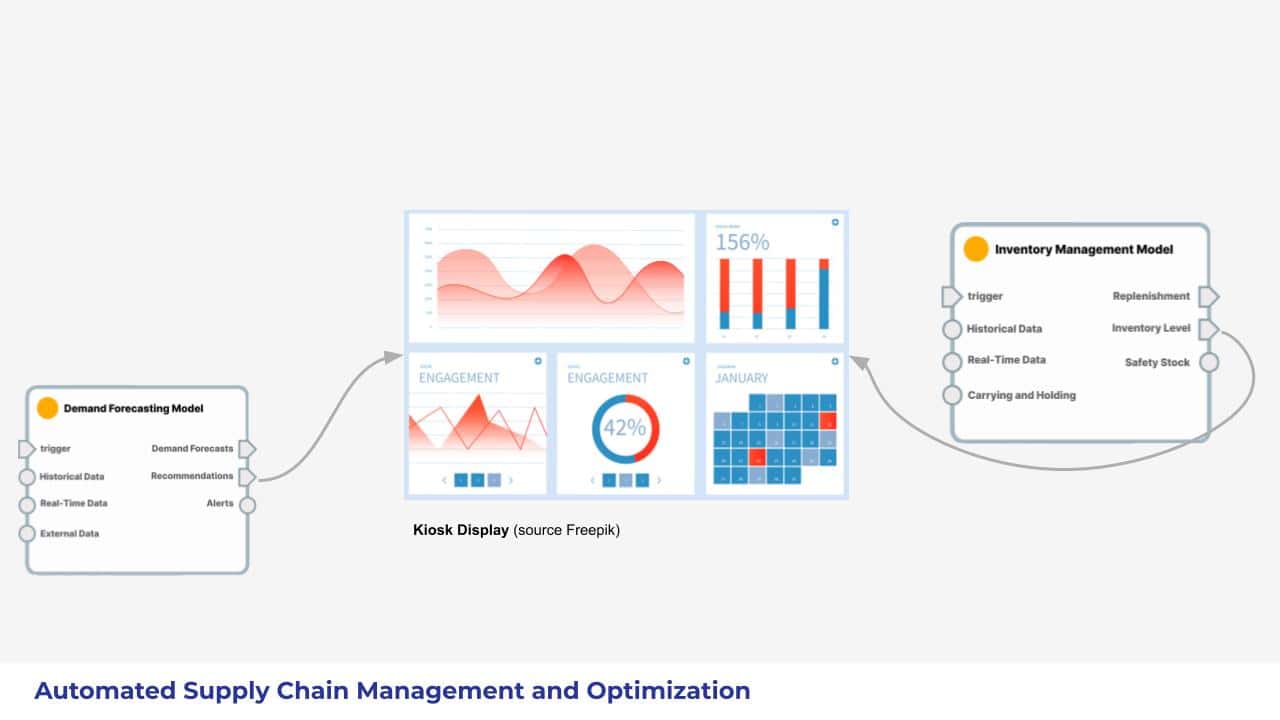
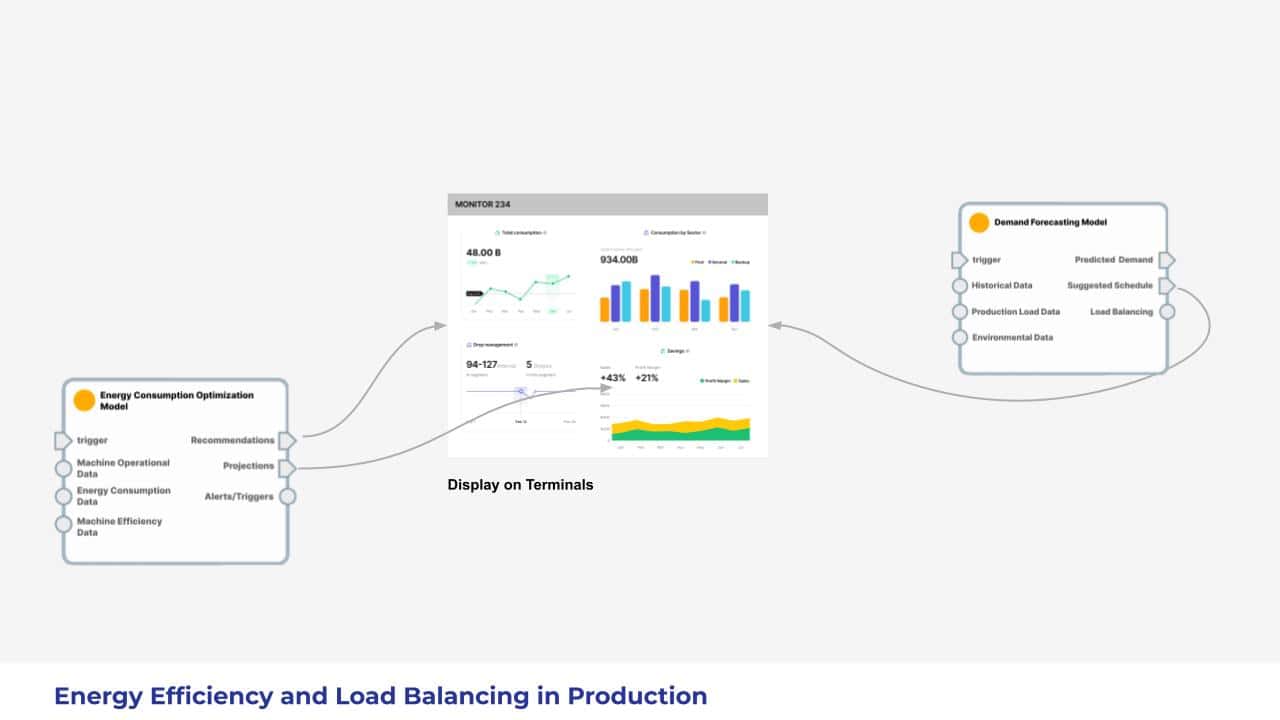
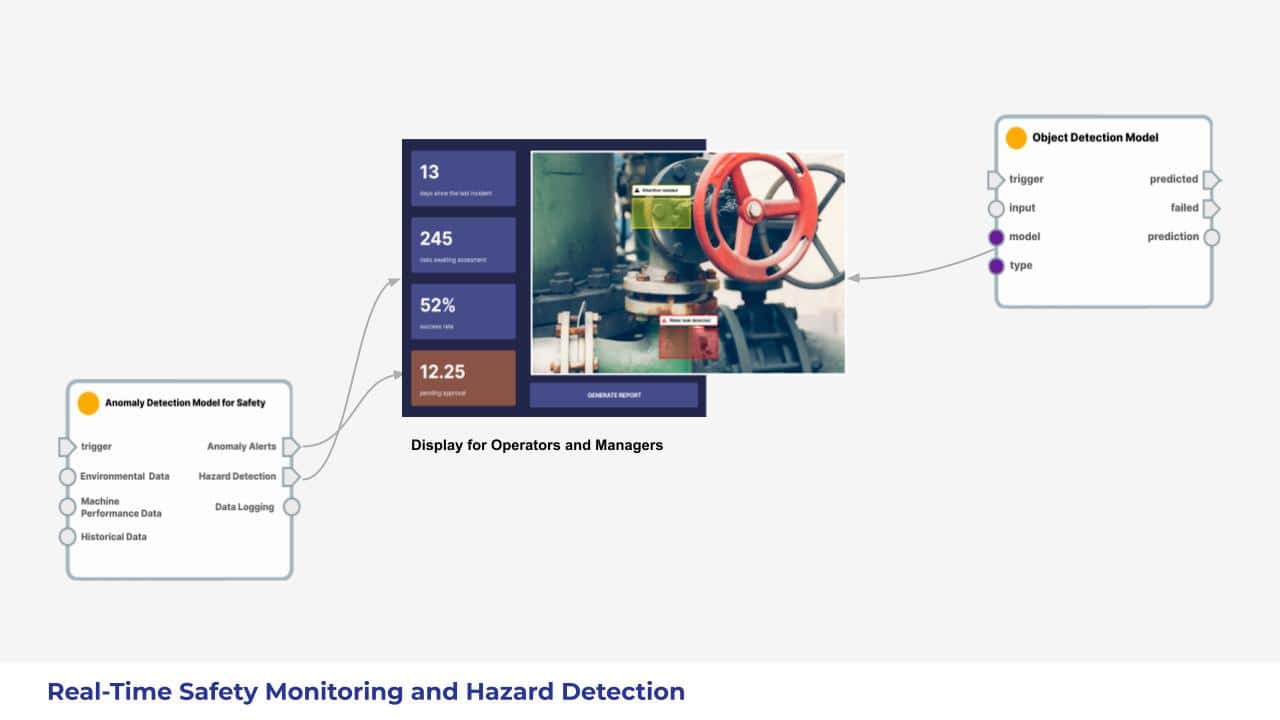
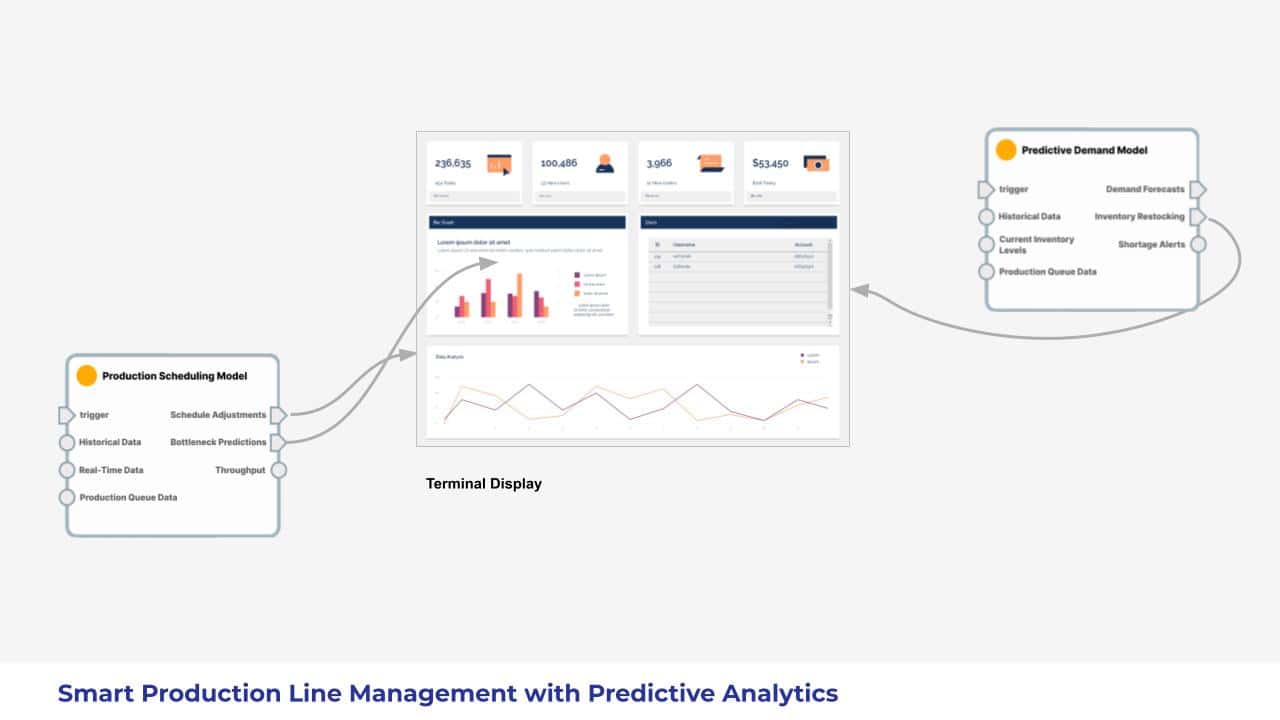

Made for production, manufacturing, and beyond:
Are you ready to harness the power of Machine Learning to generate knowledge and drive your business forward? Schedule a free platform tour today.
NC-Vision is committed to helping the manufacturing industry make the most of no-code software development. We offer a range of no-code solutions that enable companies to quickly and easily create custom solutions that are tailored to their needs. Our tools allow companies to create solutions faster, more cost-effectively, and with more customization than ever before.